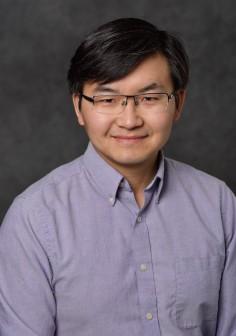
Xiaoming Liu
Michigan State University
Biometric Recognition in the Era of AI Generated Content (AIGC)
Abstract: In recent years we have witnessed impressive progress on AIGC (Artificial Intelligence Generated Content). AIGC has many applications in our society, as well as benefits diverse computer vision tasks. In the context of biometric recognition, we believe that the AIGC era calls for innovation on both data synthesis and how to leverage the synthetic data. In this talk, I will present a number of efforts that showcases these innovations, including:
1) how to bridge the gap between the training data distribution and test data distribution;
2) how to generate a complete synthetic database to train face recognition models;
3) how to estimate the 3D body shape from an image of clothed human body; and
4) how to manipulate a human body image by changing its body pose, clothing style, background, and identity.
In the end, we will briefly overview other research efforts in the Computer Vision Lab at Michigan State University.Bio: Dr. Xiaoming Liu is the MSU Foundation Professor, and Anil and Nandita Jain Endowed Professor at the Department of Computer Science and Engineering of Michigan State University (MSU). He is also a visiting scientist at Google Research. He received Ph.D. degree from Carnegie Mellon University in 2004. Before joining MSU in 2012 he was a research scientist at General Electric (GE) Global Research. He works on computer vision, machine learning, and biometrics especially on face related analysis and 3D vision. Since 2012 he helps to develop a strong computer vision area in MSU who is ranked top 15 in US according to csrankings.org. He is an Associate Editor of IEEE Transactions on Pattern Analysis and Machine Intelligence. e has authored more than 200 scientific publications, and has filed 35 patents. His work has been cited over 20000 times, with an H-index of 76. He is a fellow of IEEE and IAPR.
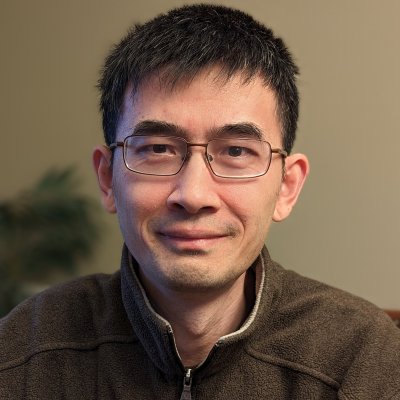
Xin Li
SUNY Albany
Aerial Insights: Recent Advances in Multiple Object Tracking and Re-Identification
Abstract: As drone technology advances rapidly, automatic analysis of aerial video has become increasingly important due to its wide applications in the real world. Multiple object tracking (MOT) and Re-identification (Re-ID) are two key techniques supporting aerial video analysis. Although different approaches have been proposed to tackle both problems, technical barriers such as abrupt scene changes and severe object occlusions have remained challenging factors. In this talk, I will survey some of the latest directions in MOT (e.g., tracking-by-detection vs. tracking-by-attention) and Re-ID (e.g., fairness detection and open-world ReID). The unifying theme advocates for a holistic approach toward object detection, tracking, and ReID and argues that aerial intelligence is a scenario where machine vision can significantly outperform human vision because humans are not airborne. I will also discuss some emerging directions at the intersection of MOT and ReID.
Bio: Dr. Xin Li received the B.S. degree with highest honors in electronic engineering and information science from University of Science and Technology of China, Hefei, in 1996, and the Ph.D. degree in electrical engineering from Princeton University, Princeton, NJ, in 2000. He was a Member of Technical Staff with Sharp Laboratories of America, Camas, WA from Aug. 2000 to Dec. 2002. From 2003 to 2023, he was a faculty member in the Lane Department of Computer Science and Electrical Engineering. Since 2023, he has been a professor in the Department of Computer Science at SUNY-Albany. Dr. Li was elected a Fellow of IEEE in 2017 for his contributions to image interpolation, restoration, and compression. Dr. Li’s research interests include computer vision, multimodal AI, computational neuroscience, and cybersecurity.
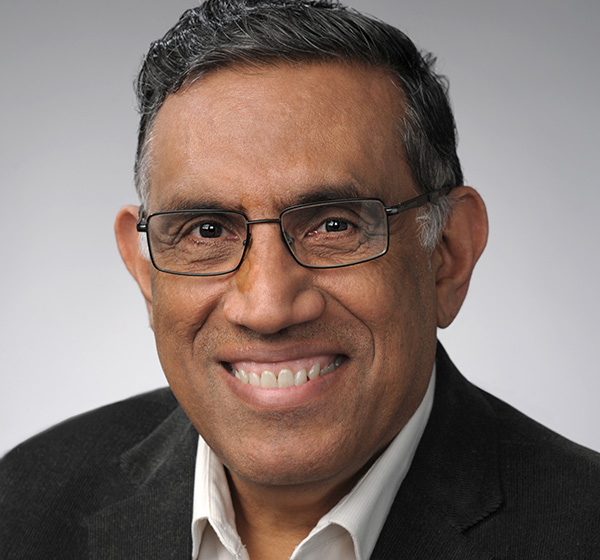
Pramod Kumar Varshney
Syracuse University
Distributed Inference in Human-Sensor Networks
Abstract: Distributed inference when the participants are only machines or electronic devices, e.g., sensors, has been explored extensively in the signal processing and machine learning literature. However, there are fundamental differences between such rational systems and systems where the agents include humans. This is due to their cognitive limitations, imperfect rationality as well as behavioral uncertainties. In information fusion systems that include humans, modeling and analysis need to take several factors into account including cognitive biases of humans, mechanisms to handle uncertainties and noise and unpredictability of humans, in contrast to inference processes consisting of only machine agents.
In this talk, we discuss some aspects of human-sensor networks by focusing on some specific problems that include collaborative human decision making, decision fusion in integrated human-machine networks, prospect theoretic human decision making in multi-agent systems and portfolio theory-based resource management. In each case, we aim to optimize the system performance based on appropriate modeling of the human behavior. We also provide a summary of current challenges and research directions related to this problem domain.
Bio: Pramod K. Varshney was born in Allahabad, India, in 1952. He received the B.S. degree in electrical engineering and computer science (with highest honors), and the M.S. and Ph.D. degrees in electrical engineering from the University of Illinois at Urbana-Champaign in 1972, 1974, and 1976 respectively. Since 1976 he has been with Syracuse University, Syracuse, NY where he is currently a Distinguished Professor of Electrical Engineering and Computer Science. His current research interests are in distributed sensor networks and data fusion, detection and estimation theory, wireless communications, machine learning, AI and radar.
Dr. Varshney was the recipient of the 1981 ASEE Dow Outstanding Young Faculty Award. He was elected to the grade of Fellow of the IEEE in 1997 for his contributions in the area of distributed detection and data fusion. In 2000, he received the Third Millennium Medal from the IEEE and Chancellor’s Citation for exceptional academic achievement at Syracuse University. He is the recipient of the IEEE 2012 Judith A. Resnik Award. He received an honorary Doctor of Engineering degree from Drexel University in 2014, ECE Distinguished Alumni Award from UIUC in 2015, the Yaakov Bar-Shalom Award for Lifetime Excellence in Information Fusion, ISIF in 2018, the Claude Shannon-Harry Nyquist Technical Achievement Award from the IEEE Signal Processing Society, the Pioneer Award from the IEEE Aerospace and Electronic Society in 2021, and Syracuse University Chancellor’s Lifetime Achievement Award in 2023.